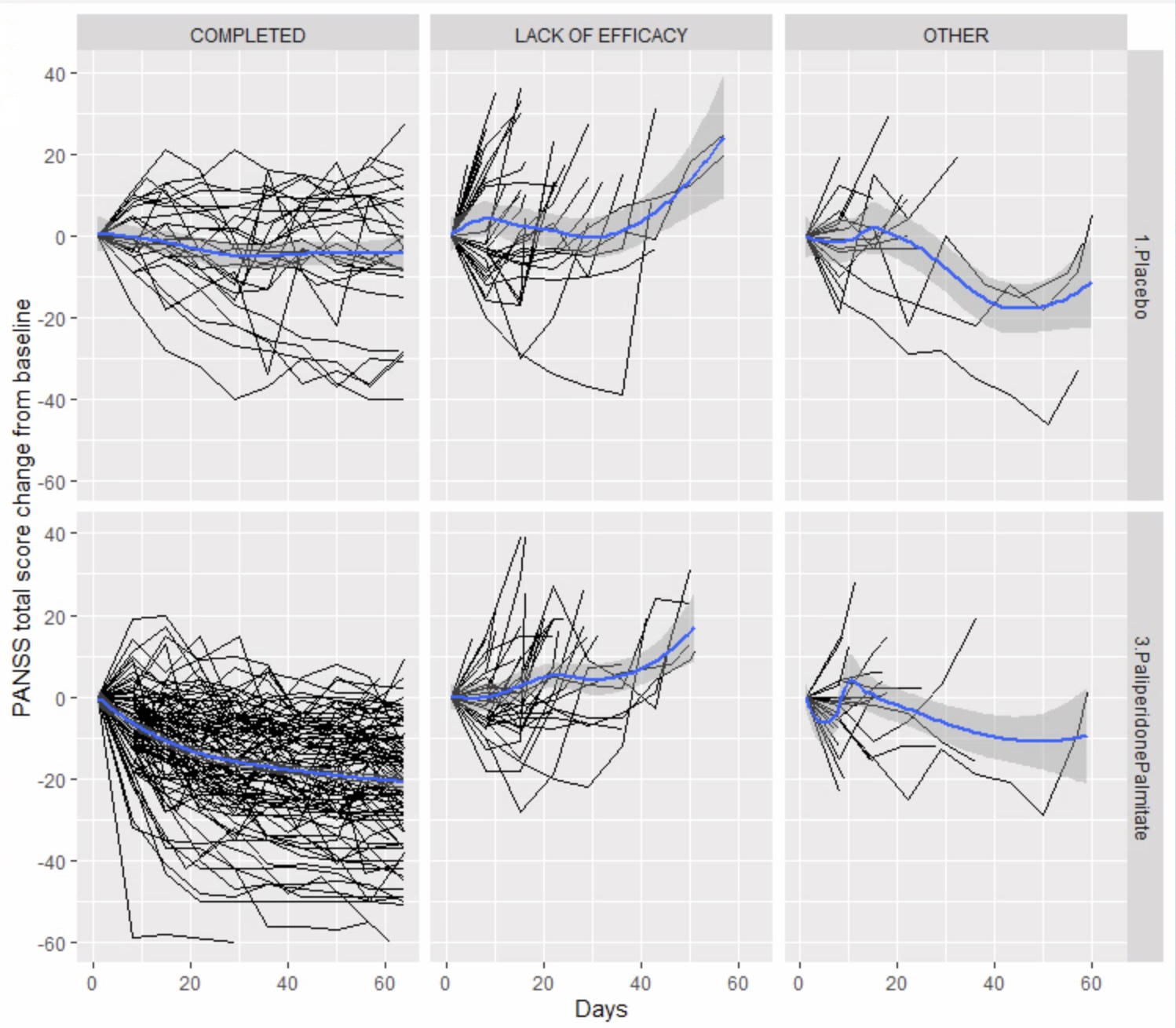
Bayesian Hierarchical Approach for Handling Non-ignorable Drop-out Across Multiple Clinical Trials in Schizophrenia
Clinical trials in Schizophrenia assess symptom severity using a clinician-rated scale like Positive and Negative Syndrome Scale (PANSS), measured over time. However, patients taking psychiatric medication have shown higher variability of response compared to patients taking medication related to a physical disorder. Within randomized trials, it has also been shown that the dropout rates can be quite large and vary between treatment groups, thus possibly introducing non-ignorable missingness or missing not-at-random (MNAR). If we combine such RCTs to evaluate treatment efficacy under individual patient-level (IPD) network meta-analysis (NMA) with non-ignorable dropout, we could be introducing bias in the estimation of the treatment effects. To address these challenges and maximize use of all available data, we aim to combine a popular method for addressing MNAR like pattern-mixture with Bayesian IPD NMA to improve the estimation of the treatment effects. Through simulations, we examine the impact of our approach under varying data availability conditions and complexity. We then apply our methods to clinical trials for schizophrenia treatments, demonstrating their effectiveness in handling non-ignorable dropout.